Hurricane intensities – Clocking hurricane wind speeds with AI models
Each day, weather satellites orbiting Earth send back thousands of images of the atmosphere and weather. These data have become an essential tool for weather predictions and tracking storms like tropical cyclones. The large volume of data and imagery produced by weather satellites each day makes the field of satellite meteorology well-suited for AI driven tasks.
In a study published in Weather and Forecasting two AI models known as D-MINT and D-PRINT demonstrated abilities to accurately predict hurricane wind speeds within 10 knots (19 kmph), sometimes exceeding the accuracy of current models used in operational forecasting.
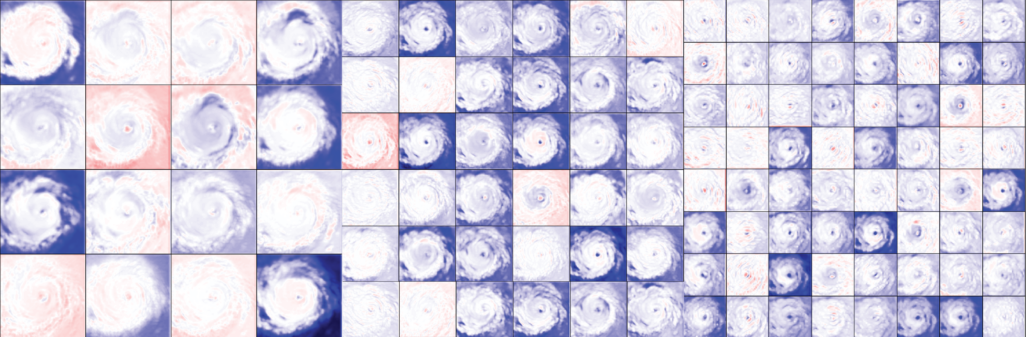
The study used more than 20 years of hurricane data and images from 1994 to 2015, providing a broad assortment of training material for the models. Convolutional Neural Network models like D-MINT and D-PRINT are capable of rapidly sifting through thousands of images to detect patterns over a hurricane’s life cycle.
Once each model had been trained to detect the patterns of more than 100 past hurricanes, they were tested on a new data from 2016-2017 to measure their accuracy at predicting wind speeds.
“Like other hurricane models, D-MINT and D-PRINT have strengths and weaknesses when it comes to predicting hurricane intensities,” says Sarah Griffin, a researcher with the University of Wisconsin-Madison Cooperative Institute for Meteorological Satellite Studies and lead author on the paper. “These AI models are better at tracking certain lower-intensity tropical cyclones compared to those used in current operational forecasting.”
The two models D-MINT and D-PRINT vary by which datasets and information they were provided. D-MINT utilized the same information as D-PRINT, however D-MINT included an extra data set using microwave satellite imagery.
Weather satellites carry a suite of instruments used to peer through Earth’s atmosphere and collect information about current atmospheric conditions. Among them are detectors sensitive to the microwave part of the electromagnetic spectrum. These can reveal useful information not provided through other channels like infrared or visible light. In the past, microwave satellite data has been used to a lesser extent in hurricane prediction than other types of data. Results from studies like this show a potential new utility for microwave data in understanding the state of the atmosphere and hurricane conditions.
Griffin says models like D-MINT and D-PRINT are best used in conjunction with other hurricane prediction models to gain a comprehensive picture of a hurricane’s development and the risk they pose to populations in their path. Other models include those developed by CIMSS scientists like the AI-Enhanced Dvorak Technique and SATCON, and those used operationally by the National Hurricane Center and the Joint Typhoon Warning Center which include the European Center for Medium-range Weather Forecasting (ECMWF) and the National Oceanic and Atmospheric Administration Global Forecast System (GFS).
Location is an important factor in hurricane prediction, and certain prediction models perform better in some parts of the world compared to others. For example, D-PRINT and D-MINT were more skillful at predicting future windspeeds for hurricanes located in the northern Indian Ocean and the western North Pacific Ocean than popular high-resolution numerical models, but less accurate than NHC predictions for the North Atlantic and eastern North Pacific regions.
Griffin says results like this can help shape the next generation of hurricane prediction tools used by forecasters worldwide and demonstrate the powerful capabilities of AI driven models.
This work is supported by CIMSS.