Improvements in radiative transfer models
Global forecast models are a go-to for meteorologists around the world as a way to predict developing weather patterns, from dangerous hurricane tracks to weekend rain or sunny skies. Since the early era of numerical weather prediction (NWP), researchers have sought to improve forecast models through refinements and validations; an evolutionary process to bolster accuracy and the speed of forecasts.
Still, there is much room for improvement.
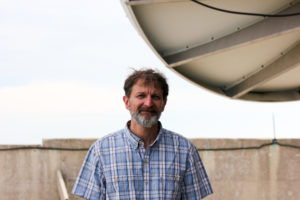
Senior CIMSS scientist Tom Greenwald is investigating ways to improve the speed and accuracy of the Community Radiative Transfer Model used in global weather forecasting models. Credit: Bill Bellon
Researcher Tom Greenwald has been working to refine forecast models for more than 10 years by focusing on the underlying mathematics and algorithms that drive the process. A senior scientist with the Cooperative Institute for Meteorological Satellite Studies (CIMSS) at the University of Wisconsin-Madison, Greenwald has partnered with Ralf Bennartz, senior scientist with CIMSS and professor, Vanderbilt University, to investigate ways to improve the speed and efficiency of what are known as radiative transfer models—models that calculate the transfer of electromagnetic radiation through the Earth’s atmosphere.
The National Centers for Environmental Prediction (NCEP) Global Forecast System (GFS) is one of several models used worldwide by meteorologists to make weather predictions. In simple terms, a model like the GFS consists of hundreds of thousands of lines of code which simulate the physics of the atmosphere and process atmospheric data from weather stations around the world and with satellite observations. At the end of the process, out comes a weather forecast.
“These models are complex,” says Greenwald. “The goal is to identify bottlenecks in the system which can slow things down and to make the process more practical from a computational standpoint.”
Greenwald is focusing his efforts on a specific area of weather modelling known as data assimilation (DA), taking satellite data and integrating it in a way that speaks the same language as the other inputs. The quality of the weather forecast depends heavily upon the quality of the input data, which creates a continuous push-and-pull relationship between the speed and accuracy of the forecasts.
Within the DA process, Greenwald is investigating ways to improve the Joint Center for Satellite Data Assimilation (JCSDA) Community Radiative Transfer Model (CRTM) (which is the link between the forecast model and satellite observations) in clouds and precipitation to speed up the process.
“Radiative transfer models used today have an established level of accuracy, but they can bog down the process,” says Greenwald. “Our research is looking at ways to use these highly accurate but slow models together with so-called ultrafast models that are less accurate, but very fast in a way that increases speed and preserves the accuracy of the results.”
The key issue in the balance of speed vs. accuracy, though, lies in the complexity of the global forecasting model itself. Swapping out one component for another is not as straightforward as replacing a lightbulb. Interdependencies within the model create a delicate balance, requiring rigorous testing and validation before implementing new algorithms to ensure errors are not compounded down the line.
Greenwald has identified three areas within the process to locate compatibilities and improve the accuracy and efficiency of radiative transfer outputs:
- Determine if radiative “scattering” is needed in the first place. In many instances, atmospheric conditions can be used in a way that allows a forecast model to save on computational resources and ignore this step.
- Improvements in the understanding of errors in scattering atmospheres. Approximations in the scattering properties of cloud and precipitation particles, the common assumption of using a single radiative transfer calculation per instrument channel, as well as ignoring three-dimensional cloud structure all contribute to uncertainties in radiative transfer calculations.
- Reducing model errors. For instance, the use of more precise cloud and precipitation scattering properties based on microphysics that are consistent with those assumed in the forecast model can help to further reduce errors.
Greenwald is looking to improve the efficiency of how to bring together satellite observations of clouds and precipitation with forecast models in DA, since these observations are expected to improve weather forecasts but are not yet fully utilized in US operational forecasting, he says.
“Our research has had a direct impact on this connection in the past and will continue to enable the greater use of satellite observations in these conditions,” says Greenwald.
Following the first year of the study, Greenwald plans to start the second year investigating the impact of the CRTM improvements on the NASA Goddard Earth Observing System Model, Version 5 (GEOS-5) data assimilation system, as well as the NOAA Global Data Assimilation System (GDAS).
This work is supported by the JCSDA and NASA Grant: Optimizing Multiple Scattering Calculations in the CRTM Grant number: NNX17AG64G
by Eric Verbeten
Cover image: