Strong showing by SSEC scientists at annual severe weather experiment
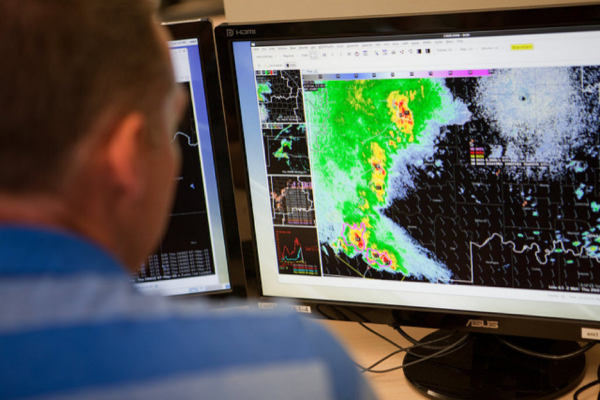
ProbSevere, a statistical storm-prediction model developed at SSEC, has been tested the past three years at NOAA’s Hazardous Weather Testbed in Norman, Oklahoma. Several SSEC scientists attended the testbed’s Spring Experiment this year. Credit: NOAA.
Each spring, at the height of severe weather season in the Midwest, weather forecasters and researchers from all over the country congregate at the Hazardous Weather Testbed – a National Oceanic and Atmospheric Administration (NOAA) joint facility managed by the National Severe Storms Laboratory (NSSL), the Storm Prediction Center (SPC), and the National Weather Service (NWS) Weather Forecast Office in Norman, Oklahoma – to test the latest and greatest in severe weather prediction technologies.
The month-long event, called the Spring Experiment, fosters hands-on, collaborative evaluation of up-and-coming platforms and techniques. By streamlining research-to-operations communication, attendees hope to ultimately improve NWS forecasts and warnings – providing critical guidance to the public during severe weather events.
“It’s my favorite trip of the year, every year,” says Justin Sieglaff, a scientist at the Space Science and Engineering Center (SSEC) at the University of Wisconsin-Madison.
Sieglaff co-leads development on ProbSevere, an experimental statistical model that predicts the likelihood of a storm producing severe weather in a 60-minute window. The model, developed by Sieglaff, SSEC scientist John Cintineo, and Mike Pavolonis, a NOAA scientist based at SSEC, has been evaluated at the Spring Experiment the past three years (and is currently in operation at more than 50 NWS offices across the U.S. for real-world weather prediction).
This year’s experiment, plus a concurrent GOES-R and Joint Polar Satellite System (JPSS) satellite proving ground experiment, ran from April 18-May 13. The experiments were scheduled to coincide with Super Rapid Scan Operations for GOES-R (SRSOR) operations – a special training period during which the current U.S. geosynchronous satellite (GOES-14) collects one-minute data to simulate the imagery capabilities of next-generation satellite GOES-R, set to launch in October 2016.
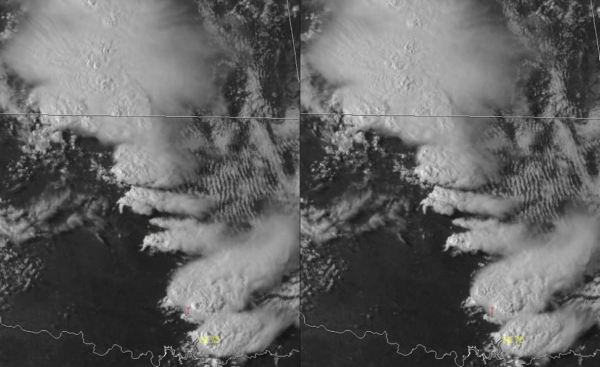
GOES-14 high time resolution imagery (left) and GOES-14 imagery shown less frequently (right). Click to view animation. Credit: Tim Schmit, SSEC.
Attendees were able to test an exciting new feature of ProbSevere for the first time: total lightning observations. Comprising cloud-to-ground and inter-cloud lightning observations from a ground-based network, the feature significantly improved the model’s performance.
“Including total lightning helped our skill measures and our lead time went up a little bit,” reports Sieglaff — the lead time is the amount of time between a severe weather event and its warning.
Developed last winter, the feature promises to have a number of scientific benefits – further accentuated by the advent of GOES-R, which will have a lightning mapping array.
“These will be observations from geostationary orbit that no one has had before,” Sieglaff says.
This was the first year that Pavolonis, the NOAA principal investigator for ProbSevere, could see the model in action at the Spring Experiment.
“To see a problem through the forecaster’s eyes is illuminating,” he says. “They are the ones who have to make that yes-no decision very, very quickly. Getting insight into how they do that, the process they have, is very useful. It helps guide us in our research to make products that are tailored to the job they have to do. It was great to get exposure to that.”
This year also marks the beginning of ProbSevere’s integration into another, newer component of the Spring Experiment: the Probabilistic Hazard Information (PHI) component of the Experimental Warning Program (EWP). The PHI is an early step toward a future severe-weather warning paradigm proposed by NOAA/NWS, in which various probabilities would be assigned within a polygon to issue more geographically nuanced severe weather warnings.
Traditionally, in the current warning paradigm, forecasters create a polygon superimposed on a map indicating that severe weather might occur within that region. But in the proposed paradigm, colorful swaths inside the polygon would indicate varying probabilities of severe weather occurring.
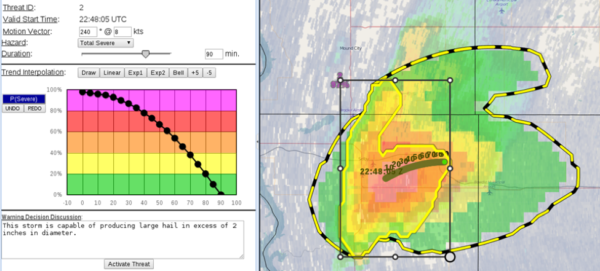
The ProbSevere model is heavily leveraged to generate probabilistic forecasts for severe weather hazards, such as large hail, being tested as part of the Experimental Warning Program. Credit: NOAA.
“You could look at that and say, ‘Am I at the 10% part of the polygon, or in the 90% part?’ It allows people to make better decisions of how to react to a potential hazard that might be coming,” Pavolonis says.
This could give emergency managers more time to react and plan for a potentially dangerous situation, he explains.
Back at the HWT, ProbSevere is currently being used to help generate those next-generation probability swaths, automatically, for the first time ever.
“It’s still experimental, but this is the first real result showing that this could work,” he says.
One of the most notable aspects of the Spring Experiment is how realistic everything is made to seem. Attendees are set up at top-of-the-line workstations, using the tools in real time with simulated severe weather scenarios. This year’s experiment, with the help of the GOES-R SRSOR one-minute imagery, investigated several high-impact environmental phenomena: major flooding in Houston, Texas; a significant hail storm in the Washington D.C. area, and the Fort McMurray wildfire in Alberta, Canada.
Forecasters are encouraged to provide feedback directly to the products’ developers to help improve functionality and design, and end each day with a wrap-up webinar called “Tales from the Testbed.” Many attendees, including Sieglaff and Cintineo, also blog about their experiences, providing an in-the-moment record of their activities.
“We are always seeking feedback,” says Sieglaff, stating that the Spring Experiment is “unique in that, as a researcher, we can sit down with people in operations, people who will actually use our research, and work with them one-on-one for an extended period of time.”
Some of the most “valuable insights from forecasters come from off-the-cuff conversations,” he adds.
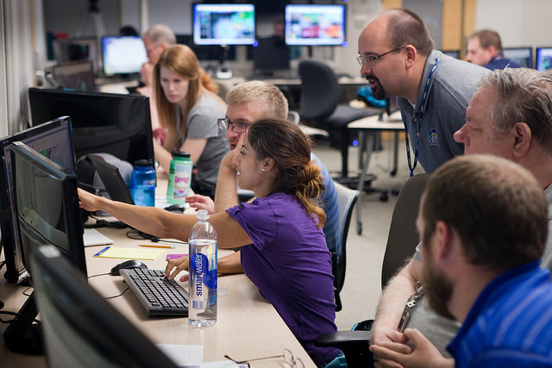
SSEC/CIMSS scientist Justin Sieglaff at the Hazardous Weather Testbed (HWT) Spring Experiment. Credit: NOAA.
Not only does the team seek feedback on the science, says Sieglaff, but the design is equally important: People are more likely to use a product with an attractive, uncluttered, functional display. SSEC scientists Jordan Gerth and Lee Cronce provide support for ProbSevere’s display using the Advanced Weather Interactive Processing System (AWIPS).
In addition to ProbSevere, two other products developed by SSEC scientists were evaluated at the testbed this year.
Firstly, the one-minute imagery from GOES-14 SRSOR for the experiment was led by Tim Schmit, a NOAA scientist based at SSEC; Dan Lindsey and Steve Goodman from NOAA’s National Environmental Satellite, Data, and Information Service (NESDIS); and Bob Rabin, an SSEC scientist at NOAA’s National Severe Storms Laboratory. This imagery allows forecasters to observe atmospheric features and processes with greatly heightened timeliness – which, when implemented in an operations setting, will translate to improved severe weather advisories.
“Getting the experimental one-minute scan GOES imagery in front of the forecasters has been critical for preparing for the rapid scan mode that will be operational on the next generation geostationary imagers,” says Schmit.
Also, SSEC scientist Jun Li is the principal investigator for a blended, all-sky product that combines three elements: the GOES-R Legacy Atmospheric Profile (LAP) operational retrieval algorithm for GOES Sounder data in clear skies, the GOES-R risk-reduction retrieval algorithm for GOES Sounder data in cloudy skies, and the Global Forecast System Numerical Weather Prediction (NWP) model in cloudy skies without successful retrievals.
LAP, as the product is commonly called, provides all-sky details about the recent evolution of moisture and instability in the atmosphere, which are important factors during pre-storm mesoscale analysis. It also has the ability to increase forecasters’ confidence regarding the state of the current thermodynamic environment.
While NWP models are limited in the information they can provide regarding atmospheric instability and moisture transport – two important pieces of the puzzle for forecasters trying to better understand a developing storm – the new generation of geostationary satellites can provide reliable data every 10 minutes. The LAP product is a helpful way to extract meaning from those data.
“The algorithmic methodology our team has developed is able to immediately translate that information so that forecasters can use it effectively and efficiently to assist issuing warnings in a timely manner,” Li says.
In terms of the GOES-R/JPSS proving ground, ProbSevere helps demonstrate the risk-reduction capabilities of the next-generation satellite, while LAP helps demonstrate both risk-reduction and baseline capabilities. Schmit and Rabin’s one-minute imagery does the latter.
Overall, says Schmit, “the HWT is a great laboratory to get feedback from operational forecasters, both government and broadcast meteorologists, related to experimental products.”
by Sarah Witman